Artificial Intelligent (AI) is a wide-ranging branch of computer science concerned with building smart machines capable of performing tasks that typically require human intelligence. AI is an interdisciplinary science with multiple approaches, but advancements in machine learning and deep learning are creating a paradigm shift in virtually every sector of the tech industry.
Artificial intelligence allows machines to model, and even improve upon, the capabilities of the human mind. From the development of self-driving cars to the proliferation of smart assistants like Siri and Alexa, AI is a growing part of everyday life. As a result, many tech companies across various industries are investing in artificially intelligent technologies.
Introduction
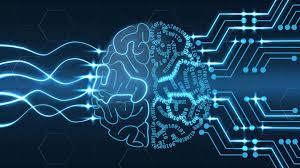
Less than a decade after helping the Allied forces win World War II by breaking the Nazi encryption machine Enigma, mathematician Alan Turing changed history a second time with a simple question: “Can machines think?”
Turing’s 1950 paper “Computing Machinery and Intelligence” and its subsequent Turing Test established the fundamental goal and vision of AI.
At its core, AI is the branch of computer science that aims to answer Turing’s question in the affirmative. It is the endeavor to replicate or simulate human intelligence in machines. The expansive goal of AI has given rise to many questions and debates. So much so that no singular definition of the field is universally accepted.
The major limitation in defining AI as simply “building machines that are intelligent” is that it doesn’t actually explain what AI is and what makes a machine intelligent. AI is an interdisciplinary science with multiple approaches, but advancements in machine learning and deep learning are creating a paradigm shift in virtually every sector of the tech industry.
However, various new tests have been proposed recently that have been largely well received, including a 2019 research paper entitled “On the Measure of Intelligence.” In the paper, veteran deep learning researcher and Google engineer François Chollet argues that intelligence is the “rate at which a learner turns its experience and priors into new skills at valuable tasks that involve uncertainty and adaptation.” In other words: The most intelligent systems are able to take just a small amount of experience and go on to guess what would be the outcome in many varied situations.
Meanwhile, in their book Artificial Intelligence: A Modern Approach, authors Stuart Russell and Peter Norvig approach the concept of AI by unifying their work around the theme of intelligent agents in machines. With this in mind, AI is “the study of agents that receive percepts from the environment and perform actions.”
What are the applications of Artificial Intelligent?
Artificial intelligence has made its way into a wide variety of markets. Here are nine examples.
AI in healthcare: The biggest bets are on improving patient outcomes and reducing costs. Companies are applying machine learning to make better and faster diagnoses than humans. One of the best-known healthcare technologies is IBM Watson. It understands natural language and can respond to questions asked of it. The system mines patient data and other available data sources to form a hypothesis, which it then presents with a confidence scoring schema. Other AI applications include using online virtual health assistants and chatbots to help patients and healthcare customers find medical information, schedule appointments, understand the billing process and complete other administrative processes. An array of AI technologies is also being used to predict, fight and understand pandemics such as COVID-19.
AI in business: Machine learning algorithms are being integrated into analytics and customer relationship management (CRM) platforms to uncover information on how to better serve customers. Chatbots have been incorporated into websites to provide immediate service to customers. Automation of job positions has also become a talking point among academics and IT analysts.
AI in education: AI can automate grading, giving educators more time. It can assess students and adapt to their needs, helping them work at their own pace. AI tutors can provide additional support to students, ensuring they stay on track. And it could change where and how students learn, perhaps even replacing some teachers.
AI in finance: AI in personal finance applications, such as Intuit Mint or TurboTax, is disrupting financial institutions. Applications such as these collect personal data and provide financial advice. Other programs, such as IBM Watson, have been applied to the process of buying a home. Today, artificial intelligence software performs much of the trading on Wall Street.
AI in law: The discovery process — sifting through documents — in law is often overwhelming for humans. Using AI to help automate the legal industry’s labor-intensive processes is saving time and improving client service. Law firms are using machine learning to describe data and predict outcomes, computer vision to classify and extract information from documents and natural language processing to interpret requests for information.
AI in manufacturing: Manufacturing has been at the forefront of incorporating robots into the workflow. For example, the industrial robots that were at one time programmed to perform single tasks and separated from human workers, increasingly function as cobots: Smaller, multitasking robots that collaborate with humans and take on responsibility for more parts of the job in warehouses, factory floors and other workspaces.
AI in banking: Banks are successfully employing chatbots to make their customers aware of services and offerings and to handle transactions that don’t require human intervention. AI virtual assistants are being used to improve and cut the costs of compliance with banking regulations. Banking organizations are also using AI to improve their decision-making for loans, and to set credit limits and identify investment opportunities.
AI in transportation: In addition to AI’s fundamental role in operating autonomous vehicles, AI technologies are used in transportation to manage traffic, predict flight delays, and make ocean shipping safer and more efficient.
Security: AI and machine learning are at the top of the buzzword list security vendors use today to differentiate their offerings. Those terms also represent truly viable technologies. Organizations use machine learning in security information and event management (SIEM) software and related areas to detect anomalies and identify suspicious activities that indicate threats. By analyzing data and using logic to identify similarities to known malicious code, AI can provide alerts to new and emerging attacks much sooner than human employees and previous technology iterations. The maturing technology is playing a big role in helping organizations fight off cyber attacks.
The Future of AI
When one considers the computational costs and the technical data infrastructure running behind artificial intelligence, actually executing on AI is a complex and costly business. Fortunately, there have been massive advancements in computing technology, as indicated by Moore’s Law, which states that the number of transistors on a microchip doubles about every two years while the cost of computers is halved.
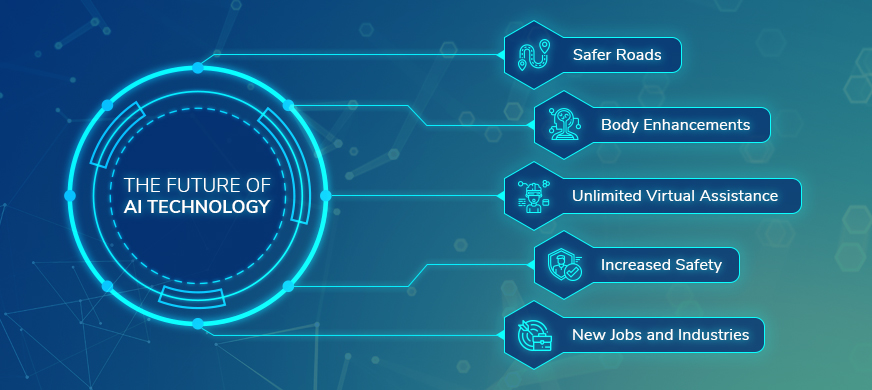
Although many experts believe that Moore’s Law will likely come to an end sometime in the 2020s, this has had a major impact on modern AI techniques — without it, deep learning would be out of the question, financially speaking. Recent research found that AI innovation has actually outperformed Moore’s Law, doubling every six months or so as opposed to two years.
By that logic, the advancements artificial intelligence has made across a variety of industries have been major over the last several years. And the potential for an even greater impact over the next several decades seems all but inevitable.
Reactive Machines
A reactive machine follows the most basic of AI principles and, as its name implies, is capable of only using its intelligence to perceive and react to the world in front of it. A reactive machine cannot store a memory and, as a result, cannot rely on past experiences to inform decision making in real time.
Perceiving the world directly means that reactive machines are designed to complete only a limited number of specialized duties. Intentionally narrowing a reactive machine’s worldview is not any sort of cost-cutting measure, however, and instead means that this type of AI will be more trustworthy and reliable — it will react the same way to the same stimuli every time.
A famous example of a reactive machine is Deep Blue, which was designed by IBM in the 1990s as a chess-playing supercomputer and defeated international grandmaster Gary Kasparov in a game. Deep Blue was only capable of identifying the pieces on a chess board and knowing how each moves based on the rules of chess, acknowledging each piece’s present position and determining what the most logical move would be at that moment. The computer was not pursuing future potential moves by its opponent or trying to put its own pieces in better position. Every turn was viewed as its own reality, separate from any other movement that was made beforehand.
Another example of a game-playing reactive machine is Google’s AlphaGo. AlphaGo is also incapable of evaluating future moves but relies on its own neural network to evaluate developments of the present game, giving it an edge over Deep Blue in a more complex game. AlphaGo also bested world-class competitors of the game, defeating champion Go player Lee Sedol in 2016.
Though limited in scope and not easily altered, reactive machine AI can attain a level of complexity, and offers reliability when created to fulfill repeatable tasks.
Limited Memory
Limited memory AI has the ability to store previous data and predictions when gathering information and weighing potential decisions — essentially looking into the past for clues on what may come next. Limited memory AI is more complex and presents greater possibilities than reactive machines.
Limited memory AI is created when a team continuously trains a model in how to analyze and utilize new data or an AI environment is built so models can be automatically trained and renewed.
When utilizing limited memory AI in ML, six steps must be followed: Training data must be created, the ML model must be created, the model must be able to make predictions, the model must be able to receive human or environmental feedback, that feedback must be stored as data, and these these steps must be reiterated as a cycle.
There are several ML models that utilize limited memory AI:
- Reinforcement learning, which learns to make better predictions through repeated trial and error.
- Recurrent neural networks (RNN), which uses sequential data to take information from prior inputs to influence the current input and output. These are commonly used for ordinal or temporal problems, such as language translation, natural language processing, speech recognition and image captioning. One subset of recurrent neural networks is known as long short term memory (LSTM), which utilizes past data to help predict the next item in a sequence. LTSMs view more recent information as most important when making predictions, and discount data from further in the past while still utilizing it to form conclusions.
- Evolutionary generative adversarial networks (E-GAN), which evolve over time, growing to explore slightly modified paths based off of previous experiences with every new decision. This model is constantly in pursuit of a better path and utilizes simulations and statistics, or chance, to predict outcomes throughout its evolutionary mutation cycle.
- Transformers, which are networks of nodes that learn how to do a certain task by training on existing data. Instead of having to group elements together, transformers are able to run processes so that every element in the input data pays attention to every other element. Researchers refer to this as “self-attention,” meaning that as soon as it starts training, a transformer can see traces of the entire data set.
Theory of Mind
Theory of mind is just that — theoretical. We have not yet achieved the technological and scientific capabilities necessary to reach this next level of AI.
The concept is based on the psychological premise of understanding that other living things have thoughts and emotions that affect the behavior of one’s self. In terms of AI machines, this would mean that AI could comprehend how humans, animals and other machines feel and make decisions through self-reflection and determination, and then will utilize that information to make decisions of their own.
Essentially, machines would have to be able to grasp and process the concept of “mind,” the fluctuations of emotions in decision making and a litany of other psychological concepts in real time, creating a two-way relationship between people and AI.
Self-Awareness
Once theory of mind can be established, sometime well into the future of AI, the final step will be for AI to become self-aware. This kind of AI possesses human-level consciousness and understands its own existence in the world, as well as the presence and emotional state of others. It would be able to understand what others may need based on not just what they communicate to them but how they communicate it.
Self-awareness in AI relies both on human researchers understanding the premise of consciousness and then learning how to replicate that so it can be built into machines.
History of Artificial Intelligence
Intelligent robots and artificial beings first appeared in ancient Greek myths. And Aristotle’s development of syllogism and its use of deductive reasoning was a key moment in humanity’s quest to understand its own intelligence. While the roots are long and deep, the history of AI as we think of it today spans less than a century. The following is a quick look at some of the most important events in AI.
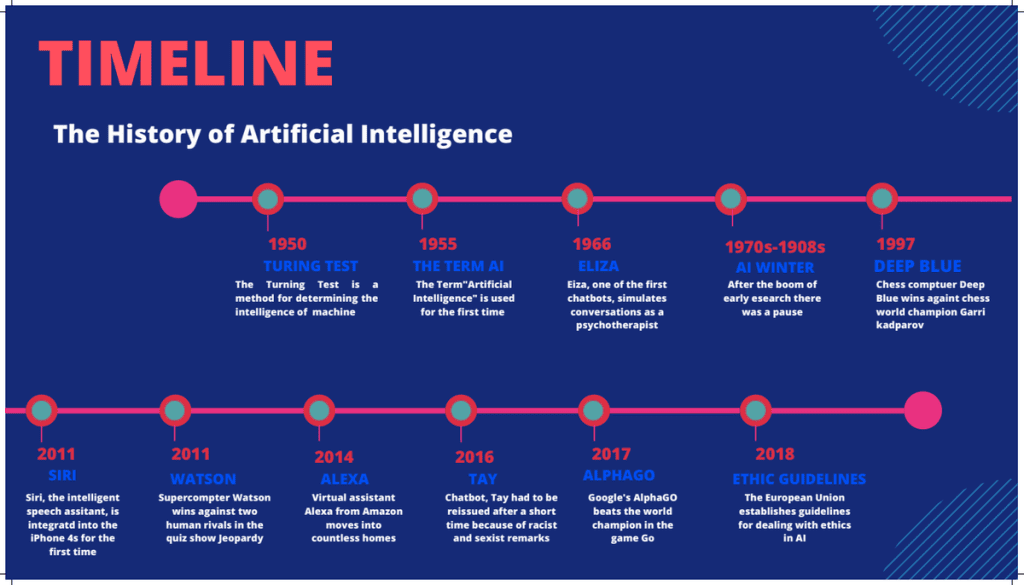
1940s
- (1943) Warren McCullough and Walter Pitts publish the paper “A Logical Calculus of Ideas Immanent in Nervous Activity,” which proposes the first mathematical model for building a neural network.
- (1949) In his book The Organization of Behavior: A Neuropsychological Theory, Donald Hebb proposes the theory that neural pathways are created from experiences and that connections between neurons become stronger the more frequently they’re used. Hebbian learning continues to be an important model in AI.
1950s
- (1942) Isaac Asimov publishes the Three Laws of Robotics, an idea commonly found in science fiction media about how artificial intelligence should not bring harm to humans.
- (1950) Alan Turing publishes the paper “Computing Machinery and Intelligence,” proposing what is now known as the Turing Test, a method for determining if a machine is intelligent.
- (1950) Harvard undergraduates Marvin Minsky and Dean Edmonds build SNARC, the first neural network computer.
- (1950) Claude Shannon publishes the paper “Programming a Computer for Playing Chess.”
- (1952) Arthur Samuel develops a self-learning program to play checkers.
- (1954) The Georgetown-IBM machine translation experiment automatically translates 60 carefully selected Russian sentences into English.
- (1956) The phrase “artificial intelligence” is coined at the Dartmouth Summer Research Project on Artificial Intelligence. Led by John McCarthy, the conference is widely considered to be the birthplace of AI.
- (1956) Allen Newell and Herbert Simon demonstrate Logic Theorist (LT), the first reasoning program.
- (1958) John McCarthy develops the AI programming language Lisp and publishes “Programs with Common Sense,” a paper proposing the hypothetical Advice Taker, a complete AI system with the ability to learn from experience as effectively as humans.
- (1959) Allen Newell, Herbert Simon and J.C. Shaw develop the General Problem Solver (GPS), a program designed to imitate human problem-solving.
- (1959) Herbert Gelernter develops the Geometry Theorem Prover program.
- (1959) Arthur Samuel coins the term “machine learning” while at IBM.
- (1959) John McCarthy and Marvin Minsky found the MIT Artificial Intelligence Project.
1960s
- (1963) John McCarthy starts the AI Lab at Stanford.
- (1966) The Automatic Language Processing Advisory Committee (ALPAC) report by the U.S. government details the lack of progress in machine translations research, a major Cold War initiative with the promise of automatic and instantaneous translation of Russian. The ALPAC report leads to the cancellation of all government-funded MT projects.
- (1969) The first successful expert systems are developed in DENDRAL, a XX program, and MYCIN, designed to diagnose blood infections, are created at Stanford.
1970s
- (1972) The logic programming language PROLOG is created.
- (1973) The Lighthill Report, detailing the disappointments in AI research, is released by the British government and leads to severe cuts in funding for AI projects.
- (1974-1980) Frustration with the progress of AI development leads to major DARPA cutbacks in academic grants. Combined with the earlier ALPAC report and the previous year’s Lighthill Report, AI funding dries up and research stalls. This period is known as the “First AI Winter.”
1980s
- (1980) Digital Equipment Corporations develops R1 (also known as XCON), the first successful commercial expert system. Designed to configure orders for new computer systems, R1 kicks off an investment boom in expert systems that will last for much of the decade, effectively ending the first AI Winter.
- (1982) Japan’s Ministry of International Trade and Industry launches the ambitious Fifth Generation Computer Systems project. The goal of FGCS is to develop supercomputer-like performance and a platform for AI development.
- (1983) In response to Japan’s FGCS, the U.S. government launches the Strategic Computing Initiative to provide DARPA funded research in advanced computing and AI.
- (1985) Companies are spending more than a billion dollars a year on expert systems and an entire industry known as the Lisp machine market springs up to support them. Companies like Symbolics and Lisp Machines Inc. build specialized computers to run on the AI programming language Lisp.
- (1987-1993) As computing technology improved, cheaper alternatives emerged and the Lisp machine market collapsed in 1987, ushering in the “Second AI Winter.” During this period, expert systems proved too expensive to maintain and update, eventually falling out of favor.
1990s
- (1991) U.S. forces deploy DART, an automated logistics planning and scheduling tool, during the Gulf War.
- (1992) Japan terminates the FGCS project in 1992, citing failure in meeting the ambitious goals outlined a decade earlier.
- (1993) DARPA ends the Strategic Computing Initiative in 1993 after spending nearly $1 billion and falling far short of expectations.
- (1997) IBM’s Deep Blue beats world chess champion Gary Kasparov.
2000s
- (2005) STANLEY, a self-driving car, wins the DARPA Grand Challenge.
- (2005) The U.S. military begins investing in autonomous robots like Boston Dynamics’ “Big Dog” and iRobot’s “PackBot.”
- (2008) Google makes breakthroughs in speech recognition and introduces the feature in its iPhone app.
2010s
- (2011) IBM’s Watson handily defeats the competition on Jeopardy!.
- (2011) Apple releases Siri, an AI-powered virtual assistant through its iOS operating system.
- (2012) Andrew Ng, founder of the Google Brain Deep Learning project, feeds a neural network using deep learning algorithms 10 million YouTube videos as a training set. The neural network learned to recognize a cat without being told what a cat is, ushering in the breakthrough era for neural networks and deep learning funding.
- (2014) Google makes the first self-driving car to pass a state driving test.
- (2014) Amazon’s Alexa, a virtual home smart device, is released.
- (2016) Google DeepMind’s AlphaGo defeats world champion Go player Lee Sedol. The complexity of the ancient Chinese game was seen as a major hurdle to clear in AI.
- (2016) The first “robot citizen,” a humanoid robot named Sophia, is created by Hanson Robotics and is capable of facial recognition, verbal communication and facial expression.
- (2018) Google releases natural language processing engine BERT, reducing barriers in translation and understanding by ML applications.
- (2018) Waymo launches its Waymo One service, allowing users throughout the Phoenix metropolitan area to request a pick-up from one of the company’s self-driving vehicles.
2020s
- (2020) Baidu releases its LinearFold AI algorithm to scientific and medical teams working to develop a vaccine during the early stages of the SARS-CoV-2 pandemic. The algorithm is able to predict the RNA sequence of the virus in just 27 seconds, 120 times faster than other methods.
- (2020) OpenAI releases natural language processing model GPT-3, which is able to produce text modeled after the way people speak and write.
- (2021) OpenAI builds on GPT-3 to develop DALL-E, which is able to create images from text prompts.
- (2022) The National Institute of Standards and Technology releases the first draft of its AI Risk Management Framework, voluntary U.S. guidance “to better manage risks to individuals, organizations, and society associated with artificial intelligence.”
- (2022) DeepMind unveils Gato, an AI system trained to perform hundreds of tasks, including playing Atari, captioning images and using a robotic arm to stack blocks.
Augmented intelligence vs. artificial intelligence
Some industry experts believe the term artificial intelligence is too closely linked to popular culture, and this has caused the general public to have improbable expectations about how AI will change the workplace and life in general.
- Augmented intelligence: Some researchers and marketers hope the label augmented intelligence, which has a more neutral connotation, will help people understand that most implementations of AI will be weak and simply improve products and services. Examples include automatically surfacing important information in business intelligence reports or highlighting important information in legal filings.
- Artificial intelligence: True AI, or artificial general intelligence, is closely associated with the concept of the technological singularity — a future ruled by an artificial superintelligence that far surpasses the human brain’s ability to understand it or how it is shaping our reality. This remains within the realm of science fiction, though some developers are working on the problem. Many believe that technologies such as quantum computing could play an important role in making AGI a reality and that we should reserve the use of the term AI for this kind of general intelligence.
Popular AI cloud offerings include the following:
Computer – KnowledgeSthali